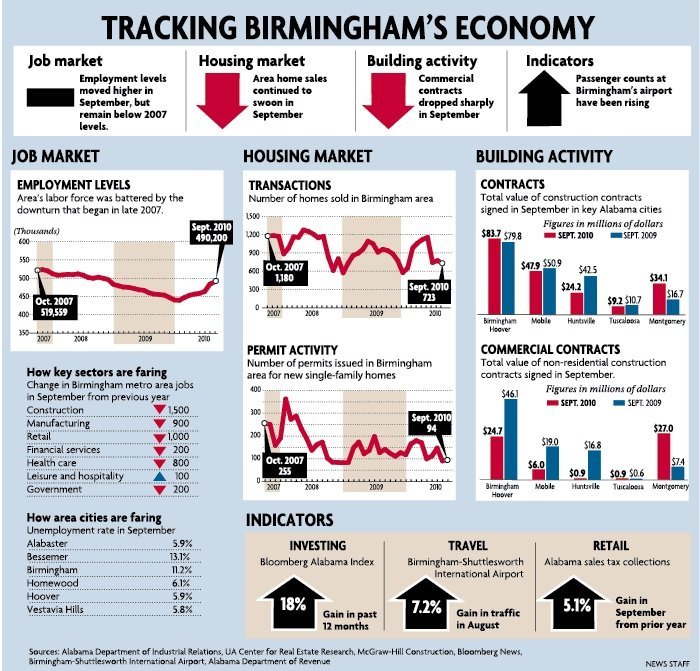
I attended the Constructing Financial Risk: Key Perspectives and Debates workshop which took place on the 13th April at CASS Business School. I was interested in learning different conceptualisations of risk as the workshop brought together experts in the subject working along different disciplines. A range of different interesting topics and relevant to Dashboards unfolded as part of the workshop including how sources and attributions of risk are delineated; the construction of risk objects (Hilgartner); the emergence of risk cultures as a ‘matter of concern’ (Power); and the reformulation of risk when algorithmic errors unfold (Millo).
Inspired by some of the ideas presented at this workshop, I want to explore a range of devices and techniques whose function has been to close the gap between what public measures are oriented towards signalling and indicating and how citizens experience, perceive but also become acquainted with the phenomena that such measures are particularly configured to indicate. The problem such a gap presents is also one of risk –maybe more specifically of expectations and dispositions towards the future – and one that has required to be governed and controlled.
The first example I want to draw on is the Personal Inflation Calculator. The Personal Inflation Calculator was developed by the Office for National Statistics in the UK in 2007. Originally this tool was developed in order to educate citizens on why their personal experience and perception of inflation appeared different to the one signalled by the publication of the Consumer Price Index (CPI) in the country. The ONS stated that
[…] Public perception of inflation tends to differ from official figures such as the CPI and RPI. A possible consequence of such a divergence could be a reduction in public confidence in official inflation figures. It could also impact on inflation expectations and lead to a disconnection between prices and wages. While the purpose of the CPI or the RPI is not to measure public perceptions of inflation, it is important to develop ways to explain the gap between perceived inflation rates and official measures of inflation.(2011, 1)
The gap between what official measures signal and how citizens experience the phenomenon such measures signal – such as inflation – is an unexpected and undesired effect on how inflation measures are constituted (based on averages); how they become publicised (as a singular and aggregated percentage); and also how often they become publicised (often quarterly or monthly in the case of CPI). One of the things I learned as part of the risk workshop I attended was that critical accounting researchers have identified and conceptualised this phenomenon since at least 1956 when a paper by V. F. Ridgway was published on the dysfunctional consequences of performance measurements. This is an interesting area of literature that we plan to engage with as part of our research on Dashboards. Measures, their publicity and display can create a series of risks and a range of different tools are being designed to control for risks associated with their publication. Controlling and designing technologies to close the gap that the publication of CPI produces between official inflation accounts and experiences of inflation is one of many mechanisms for reducing the risk of self-fulfilling inflationary periods occurring. In other words, PIC is a mechanism for managing inflation expectations, one which was also designed to align non-average citizens with official inflation figures.
Another interesting mechanism for reducing risk and controlling for the undesired effects of numbers has been described by Emmanuel Didier in his historical account on the making of economic statistics public in America (2005). Didier (2005) argued that the mechanisms and practices through which economic statistics in America were made to become public served to control for the unexpected or undesired effects of these numbers. As noted by Didier (2005), it was because the design of American economic statistical disclosure policy enabled synchronicity and symmetrical distribution that some forms of financial speculation were to be avoided.
These examples not only point to the reactivity of measures but to the mecnahsims, devices and techniques being deployed to contain their effects and reduce or avoid the risks that the circulation and publication of measures bring about. In recent times, dashboards have become a prominent device for making statistics public, but also for repurposing and reordering public statistics pertaining to a range of sources. A range of national and international initiatives have promoted publicising previously singular and traditionally text-based released official statistics or indicators in dashboard formats instead. It has been reported that the National Bureau of Statistics of the People’s Republic of China is soon preparing to release Gross Domestic Growth (GDP) figures as part of a dashboard containing more than forty economic indicators in order to signal the efficiency and quality of the country’s economic growth. In the United Kingdom the Office of National Statistics (ONS) has also recently started releasing GDP figures in a dashboard design ‘for the purpose of assessing changes in the various dimensions of economic wellbeing’ (ONS 2014, 1). In America, the Federal Reserve Bank of Atlanta has developed a publicly accessible inflation dashboard to account for long-term trends in inflation measures and capture and display price variation movements from a variety of perspectives. The World Bank, the Organisation for Economic Cooperation and Development, and the World Trade Organisation are among many other local, national and international bodies which are designing and deploying dashboards as a means towards repurposing and re-presenting governmental economic indicators and statistics.
This brings I think a series of interesting questions with regards to what dashboards do to numbers but also how they might configure risk and enact the gap between expectations and official measures differently, a series of questions that we are starting to engage with as part of our Dashboard project. So what are the risks poised and the unexpected or dysfunctional outcomes of economic statistics becoming increasingly disclosed in dashboard displays? What difference does it make for the configuration of an observation economy of public numbers that these are becoming published as part of dashboards? And what is the difference in the effect being produced and the risk constructed between the intervention of a singular number intermittently in time as opposed to the continuous stream of public numbers being delivered by particular forms of display?